In People Analytics - "Context is King"
- guy brahms
- Nov 25, 2020
- 5 min read
Your organizational data tells thousands of deeply insightful stories, but you must work hard to uncover them.

Analytics is usually about numbers, metrics and charts. But when it comes to People Analytics, it's also about stories.
In an organization with hundreds or thousands of people, countless storylines and narratives exist. Every employee, contractor or intern experiences the organization a bit differently, has a different timeline and therefore different expectations: be it the unique sequence of events throughout their career in the company; their changing peers, managers, employees and co-workers; their personal circumstances; or other external factors. All these factors could be summed up into one word: Context. To paraphrase Bill Gates - when it comes to people analytics, Context is king. Understanding and analyzing the context of employees, and not just their current “state”, is essential for organizations who wish to create a more personal and nuanced relationship with their people and drive better results all around.
Enter People Analytics.
More and more organizations around the world are trying to utilize the data stored in their different information systems, or to collect new data, to form a better understanding of their workforce. And rightly so - the data a modern organization possesses has the potential to unlock immensely valuable insights. By combining data from different domains (demography, organizational structure, compensation, engagement, finance and many more), organizations can uncover a holistic picture of their employees' experience at the company like never before.
However, uncovering meaningful stories from the data and making them actionable is no easy task. One of the major barriers that must be overcome is – rather surprisingly – the data itself.
The problem with static data
Log in to a common information system storing organizational people data, and right away you'll find yourself facing context's worst enemy: static data.
As many of these systems were built to support transactional activity, they're just doing their jobs: helping users understand the absolute status of each person at a specific moment in time (usually that time is ‘now’). This type of "snapshot" data structure is essential for enabling organizational day-to-day activity, and obviously, it can be useful for basic analytics and reporting too (especially when aggregated). Unfortunately, the timeline and broader context are usually lost somewhere in the background or in system logs, far from the user's reach. Therefore, this type of data is missing a lot of the details in the picture.
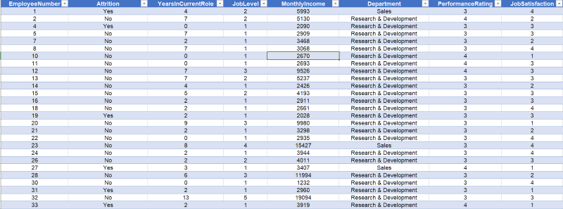
As a Co-Founder at a Predictive People Analytics startup (Manto AI), I've encountered this problem ever since founding the company. Our initial premise was that large organizations already have enough data to create explainable predictions of different business-related phenomena (in our case, we started with the business problem of undesired employee turnover). So before even touching the technological aspects of the solution, we read hundreds of academic studies conducted around the world to understand which parameters cause, correlate or predict voluntary employee turnover. Surprisingly (or not), we found that most parameters are relative and not absolute. This means that we'll probably not find them in an HR system as-is.
Let's look at some examples:
The most obvious one, which organizations are usually aware of, is compensation. Looking at the absolute salary an employee earns right now rarely teaches much. It's the relative attributes – salary relative to market benchmark; salary relative to peers within the company; salary trend (over time); and many more – that really tell a meaningful story. If you're attempting to predict voluntary turnover, for instance, it's important to understand that an employee will usually not leave the company because she's earning $10,000 a month, but because she believes she can earn much more elsewhere or because she feels she's treated unfairly in comparison to her peers.
Here's a less straightforward example: countless studies show that "career development" is a prominent cause for voluntary turnover. But what is "career development" and can you even see it in your data?
If you try using basic HR data to understand "career development", you'll probably conclude that it's impossible. But you’d be wrong. Here are just 4 out of dozens of career-development-related parameters that Manto creates from existing HR data:
Absolute and relative promotion pace (based on current and past roles, managerial levels and tenure).
Promotion and internal mobility potential (based on promotion history of relevant peers, managers or predecessors in the current role).
Professional training (based on amounts, durations, and dates of professional courses).
Job Security (based on trends in dismissal of relevant peers).
These are obviously relevant if you’re creating a predictive model on HR-related issues, but they’re interesting even on their own - wouldn’t managers do a better job if they had that information available to them? Wouldn’t HR have a better understanding of the workforce?
Help the data help you
So, what can be done?
This is the point when we roll up our sleeves and start getting our hands dirty, until we get to the holy grail – context-based data. During a process which is called "feature engineering" or more generally "data enrichment", you’ll need to put domain expertise to use, and breathe life into your static raw data until it fits the question we're trying to answer. The best tip I can give you is to always start with PETE:
Problem-specific context: for instance, If your question is related to voluntary turnover you can build parameters accordingly (what's the average separation tenure for employees in this role? What's the voluntary turnover rate under her manager and how has it changed over time? Has any of her close peers resigned during the last 3 months?)
Environment: Using data about the organizational structure (site, manager, role, team, etc.), we can extract new data about the employee's close surroundings. Examples: How big is her team and how diverse is it? How many of her peers were fired in the previous year? Is she surrounded by employees within the same profession who can help her progress? What's her mobility potential? What are the engagement survey results in her segment?
Time: Using historical data, we build time-based parameters, comparing employees to themselves over time and "extracting" events in their timeline. Examples: Did this employee experience an organizational or managerial change? How long ago? What's her promotion pace? How is her current performance faring compared to previous time periods?
External Circumstances: Using data about demographics and market trends, we can create data about the external context of the employee. Examples: What's the demand for employees with her skillset in her geographical surroundings? How does the fact that this employee has children would affect their view of their work-life balance or compensation?
I strongly encourage anyone who is serious about people analytics to dig in to context creation, and start enriching the information they’re using to produce insights to decision makers in their organization. I’m not saying it’s easy, but it’s probably the most important thing you can do with your data, and the sooner the better.
If you have any questions about how to get started - you can send me your queries to or@manto-ai.com
Comments